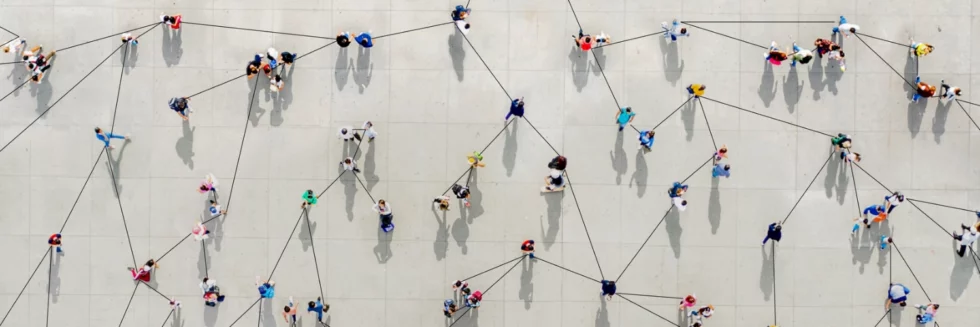
Suspicion Machines
Unprecedented experiment on welfare surveillance algorithm reveals discrimination
Governments all over the world are experimenting with predictive algorithms in ways that are largely invisible to the public. What limited reporting there has been on this topic has largely focused on predictive policing and risk assessments in criminal justice systems. But there is an area where even more far-reaching experiments are underway on vulnerable populations with almost no scrutiny.
Fraud detection systems are widely deployed in welfare states ranging from complex machine learning models to crude spreadsheets. The scores they generate have potentially life-changing consequences for millions of people. Until now, public authorities have typically resisted calls for transparency, either by claiming that disclosure would increase the risk of fraud or to protect proprietary technology.
The sales pitch for these systems promises that they will recover millions of euros defrauded from the public purse. And the caricature of the benefit cheat is a modern take on the classic trope of the undeserving poor and much of the public debate in Europe — which has the most generous welfare states — is intensely politically charged.
The true extent of welfare fraud is routinely exaggerated by consulting firms, who are often the algorithm vendors, talking it up to near 5 percent of benefits spending while some national auditors’ offices estimate it at between 0.2 and 0.4 of spending. Distinguishing between honest mistakes and deliberate fraud in complex public systems is messy and hard.
When opaque technologies are deployed in search of political scapegoats the potential for harm among some of the poorest and most marginalised communities is significant.
Hundreds of thousands of people are being scored by these systems based on data mining operations where there has been scant public consultation. The consequences of being flagged by the “suspicion machine” can be drastic, with fraud controllers empowered to turn the lives of suspects inside out.
Could journalists hold this new form of power to account? We used freedom of information laws and the courts to force disclosure of the technical details of these systems and attempted to independently assess the claims of accuracy and fairness being made on their behalf. In the process, we learned important lessons about how teams of journalists can audit a complex machine learning model and explain their findings to a general readership.
Methodology
For two years, we pursued the holy trinity of algorithmic accountability: the training data, the model file and the code for a system used by a government agency to automate risk assessments for citizens seeking government services. More than 100 FOIA requests were made across a dozen countries. We entered into correspondence and appeals processes in almost all of these places, investing scarce funds in countries like Ireland where FOIA costs are increasingly passed onto watchdogs like journalists.
Rotterdam was chosen as the centrepiece of our Suspicion Machines series not because what it is doing is especially novel, but because, out of dozens of cities we contacted, it was the only one willing to share the code behind its algorithm. Alongside this, the city also handed over the list of variables powering it, evaluations of the algorithm’s performance and the handbook used by its data scientists. And when faced with the prospect of potential court action under Europe’s equivalent to US sunshine laws — it also shared the machine learning model capable of calculating scores, providing unprecedented access.
We were able to conduct an experiment taking apart the machine learning algorithm of a risk scoring system from the inside out – rather than just analysing the inputs and outputs of the algorithm and its discriminatory patterns. This allowed us to interrogate: fundamental design choices, the entire set of input variables and assess disparate impact (See this blog for a detailed discussion of the methodology).
Every year, Rotterdam carries out investigations on some of the city’s 30,000 welfare recipients. Since 2017, the city has used a machine learning model — built with the help of consulting firm Accenture — to flag suspected welfare cheats.
Rotterdam’s fraud prediction system takes 315 inputs, including age, gender, language skills, neighbourhood, marital status and a range of subjective case worker assessments, to generate a risk score between 0 and 1. Between 2017 and 2021, officials used the risk scores generated by the model to rank every benefit recipient in the city on a list, with the top decile referred for investigation. While the exact number varied from year to year, on average, the top 1,000 “riskiest” recipients were selected for investigation. The system relies on the broad legal leeway authorities granted in the Netherlands in the name of fighting welfare fraud, including the ability to process and profile welfare recipients based on sensitive characteristics that would otherwise be protected.
It became clear that the system discriminates based on ethnicity, age, gender, and parenthood. It also revealed evidence of fundamental flaws that made the system both inaccurate and unfair.
Rotterdam’s algorithm judges people on many characteristics they cannot control, such as gender and language skills. What might appear to a caseworker to be a vulnerability, such as a person showing signs of low self-esteem, is treated by the machine as grounds for suspicion when the caseworker enters a comment into the system. The data fed into the algorithm ranges from invasive (the length of someone’s last romantic relationship) and subjective (someone’s ability to convince and influence others) to banal (how many times someone has emailed the city) and seemingly irrelevant (whether someone plays sports). Despite the scale of data used to calculate risk scores, experts say it performs little better than random selection.
These findings can be explored (in English and Dutch) with a reconstruction of Rotterdam’s welfare risk-scoring system created as part of this investigation, thanks to the Eyebeam Center for the Future of Journalism. The user interface is built on top of the city’s algorithm and demonstrates how the risk score is calculated.
Storylines
Working for six months with a team at WIRED magazine we distilled the findings from the investigation into a four-part series titled “I am not a number”. The Tech story “Inside the Suspicion Machine” gives a full narrative and interactive explanation of the inside-out audit and the context in which systems like it are deployed. The piece includes the voices of some of the world’s leading authorities on ethics and AI, including Margaret Mitchell, who explained how and why the system “is not useful in the real world” and is essentially “random guessing.”
In the People story, Lighthouse and WIRED worked with Rotterdam’s local newspaper Vers Beton to trace individuals who were flagged for investigation by the algorithm and the impact of this on their lives. Imane, a Moroccan-born, mother of two who has been the repeat subject of welfare investigations, tells of the toll on her mental and physical health, even though she has been shown to have done nothing wrong.
The Politics story travels to Denmark where a once generous welfare state has been transformed by distrust into a surveillance culture in which vast amounts of personal data from someone’s children’s travel history to machine-made guesses about who someone sleeps with are combined into fraud risk scores. An interview with Annika Jacobsen, the head of the powerful data mining unit of Denmark’s Public Benefits Administration, captures the technocratic justification for the deployment of an array of machine learning models. “I am here to catch cheaters,” she tells a reporter. “What is a violation of the citizen, really?” Jacobsen asks. “Is it a violation that you are in the stomach of the machine, running around in there?”
In reality, only 13 percent of the cases flagged by her unit are selected for further investigation by Copenhagen, the Danish capital, and human rights groups compare the data miners to the notorious National Security Agency in the US.
The Business story, ranges from the US state of Indiana to Belgrade, Serbia to portray the burgeoning “govtech” industry. It depicts an industry featuring multinationals like IBM and Accenture, as well as minnows such as the Netherlands’ Totta Data Labs and Serbia’s Saga, who trail a litany of failures, large and small.
Dutch national partner Follow the Money worked with their data team to dive into the technical details of the algorithm. They explored how fundamental design choices resulted in a model unfit for purpose. Meanwhile, radio partner VPRO Argos explored the story behind the story and the growing need for journalists to take on algorithmic accountability reporting in one of Europe’s most digitised welfare states.
To keep up to date with Lighthouse investigations sign up for our monthly newsletter