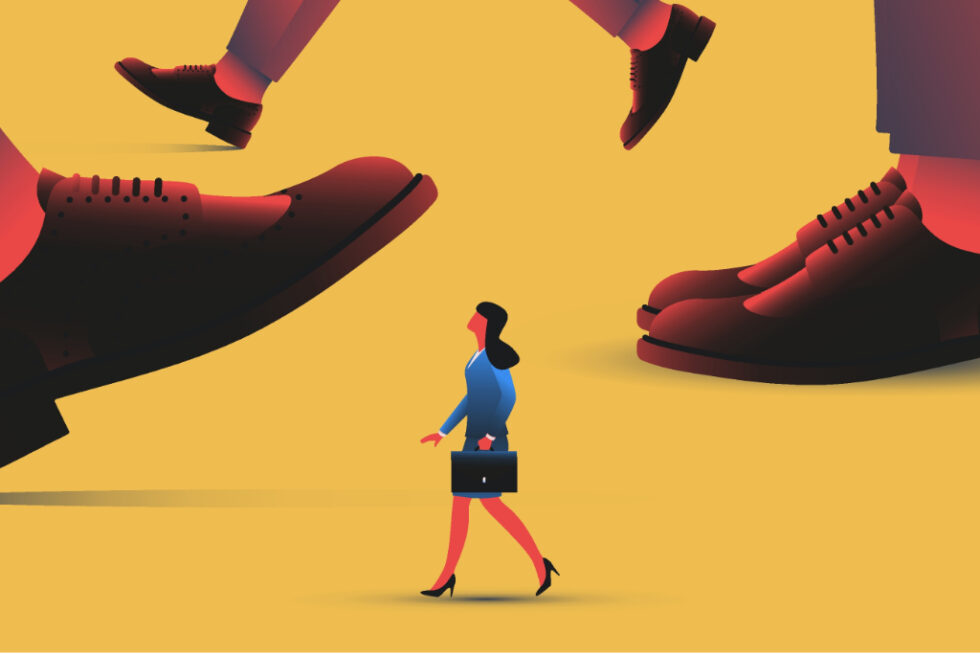
METHODOLOGY
Brain Waste Methodology
Teachers working as nannies despite overcrowded classrooms, doctors cleaning bedpans in short-staffed hospitals and engineers delivering food on motorcycles despite crumbling infrastructure. Across Europe, millions of migrants are over-qualified for jobs. This phenomenon – brain waste – leads to billions of Euros in lost wages and leaves gaping holes in many sectors already struggling with labor market shortages.
INTRODUCTION
Immigrants are crucial to European economies’ success: as European societies age, the European Commission and academics insist that to maintain economic prosperity and pay for an ever increasing number of ageing Europeans’ retirements, immigrants are needed to fill the gaping holes in many countries’ labor markets. Despite these pressing needs, millions of immigrants in Europe struggle to find employment that matches their qualifications and end up working lower paying, insecure jobs or leave the labor market altogether – a phenomenon known as ‘brain waste’. This joint investigation by Lighthouse Reports, the Financial Times, El Pais, and media partners across Europe, sets out to investigate the scale of brain waste, who is most affected, its causes, and potential solutions.
Over the past year and a half, we have become the first journalistic organization to work directly with sensitive microdata from the European Labor Force Survey (ELF), a dataset containing around 1.1 million respondents across the EEA each quarter (for more details, see ‘Data Acquisition’). Here is what we found:
– Across Europe, 47.5% of college-educated immigrants are over-qualified for their jobs compared to 32.9% of natives.
– If immigrants earned the same wages as natives, the European economy could grow by 33.8 billion Euros, or 0.36% of GDP.
– In most countries, women are more affected by brain waste than men.
– We did not find evidence that policy changes to ease the recognition of foreign diplomas substantially improved immigrants’ labor market outcomes.
– Immigrants in some fields are consistently better integrated in the labor market than others. Health care stands out as a field with relatively successful immigrant integration while the opposite is true for education.
This methodology describes the steps we took to analyze the ELF and presents the results of our analysis in interactive visualizations that invite a deeper dive into our findings. We spliced and analyzed the data in so many ways that it would be impossible to describe all interesting results here. The interactive features of the graphs are designed to enable further exploration. The datasets to construct the graphs are available by clicking the link in the description, and media partner investigations explore more granular regional and country-level findings. Our complete Github repository used for this analysis is publicly available.
DATA ACQUISITION AND ANALYSIS
We received the individual level ELF from Eurostat for all EU countries except Germany, plus Norway, Switzerland, Iceland and the United Kingdom (until 2019). The survey contains more than a hundred variables, covering respondents’ demographic background (age, gender, NUTS 2 region of residence etc.) as well as a host of labor market outcomes (employment, wages). All standard variables are contained in this codebook.
For 2008, 2014, and 2021, the ELF contains additional labor market variables specifically related to immigrants’ labor market performance, including their language skills in the host country language and whether immigrants sought and received recognition of their professional qualifications. These additional variables can be found here.
The great advantage of using the ELF is that it has been carried out using a broadly consistent methodology for all EU/EEA member states. This means we can compare how immigrants in one country are faring compared to those in another.
Because the ELF contains many sensitive variables on individuals’ labor market outcomes, we are unable to share the raw data and have to restrict ourselves to disseminating aggregated results in accordance with Eurostat’s anonymization criteria.
To supplement the ELF, we used additional data drawn from various EU agencies.
– Wages: The ELF only contains income deciles (up to 2020), i.e., it does not include the actual Euro value people are earning. We matched these deciles to annual earnings data derived from the Structures of Earnings Survey (SES), which Eurostat compiled for us. The SES dataset we received from Eurostat is accessible here.
– Regulated professions: We scraped the Regulated Professions Database directly from the website and manually matched individual professions to ISCO 2008 occupations contained in the ELF.
– Shortage occupations: We extracted the shortage occupations contained in the EURES “Report on labour shortages and surpluses”, which operates on the ISCO 2008 4-digit level. Each 3-digit occupation contained in the ELF is marked as ‘shortage’ if it contains a 4 digit occupation mentioned in the EURES report for a given country.
– GDP: We used a custom Eurostat table to derive annual GDP estimates by country. You can find the table here.
Preprocessing
Before analyzing the data, we merged the yearly datasets from 2006-2022 on the country level, derived key variables for our analysis (more details in Glossary below), merged the data with the external datasets discussed above, and restricted them to college-educated respondents between the ages of 18 and 67 only.
In addition, we merged Central/Eastern European countries into four groups: Visegrad (Czechia, Slovakia, Hungaria, Poland), the Baltic states (Estonia, Latvia, Lithuania), the 2007 EU entrants Romania and Bulgaria, and the former Yugoslav Republics of Croatia and Slovenia. We did this because in most of these countries, there are such small migrant samples (largely because there are relatively few immigrants) that sharing results would violate Eurostat’s anonymization rules.
Before merging the country-level datasets of these groups, we randomly removed respondents from countries with higher sampling rates until all countries in each group and year had the same sampling rate. We report findings on the group level but generally do not discuss the findings because of continued concerns about data reliability.
We chose to focus on college-educated respondents only because brain waste by definition is more likely to affect immigrants who received higher education that can be ‘wasted’. We chose the age boundaries for similar reasons. We used 18 as the lower age limit because respondents below 18 are unlikely to have completed any kind of tertiary education.
Where we do not explicitly mention that all respondents were included, our analysis is based on college-educated respondents only.
Analysis
Most of our findings are simple averages for various subgroups of ELF respondents. For example, to calculate the share of over-qualified migrants, we would restrict the dataset to immigrants only (or group the dataset by migration status) and then take the mean of our ‘over-qualified’ variable. Rows which contain missing data are automatically removed from such calculations. Quite often we are interested in the differences between two or more of those subgroups, especially immigrants and natives. To calculate the differences, we simply subtract one mean from another.
The problem with such “raw differences” is that migrant-native differences in labor market outcomes could be caused by a factor other than the migration background. To account for such ‘confounders’, we use linear regression analysis (OLS). This statistical analysis technique allows us to determine the impact of migration status while keeping other variables such as gender, age, educational background, etc. constant. In other words, when we use regression analysis, we no longer just compare migrants and natives, but instead we compare migrants and natives of the same gender, age, educational background, etc.
One added benefit of regression analysis is that it allows us to determine the likelihood that any observed difference is due to chance – the so-called ‘p-value’. If the ‘p-value’ is smaller than 5% – in other words, if the probability that the observed difference is a fluke is less than 5% – we call the result ‘significant’.
One important note of caution: migrants differ from natives in many important ways that can affect labor market outcomes. Even after controlling for many potential confounders, it is still possible that the differences in labor market outcomes we describe below are not caused by migration status but instead by some other variable we don’t observe in our data or one that we failed to control for. We discuss many of these potential explanatory variables in ‘The Causes of Brain Waste’ section. We do not consistently control for these variables when trying to assess the magnitude of brain waste because it was important to us to distinguish between quantifying the scale of the phenomenon and potential causes for it.
GLOSSARY
– Brain waste: The non-recognition of the skills and qualifications acquired by a migrant outside of the EU, which prevents them from fully using their potential. Definition derived from the European Commission.
– College-educated: Completed post-secondary education. Corresponds to ISCED levels 5, 6, 7, and 8.
– Migrants: Born outside the country.
– Natives: Born in the country.
– Global South: Africa, Asia, Latin and Central America, third country nationals (TCN).
– Global North: Europe (including Eastern Europe), North America, Oceania.
– Degree recognition: Foreign degrees being recognized, usually to work in a regulated profession. An immigrant has received degree recognition when they applied for and received a certificate that equivalates their foreign degree to a native one. We only use this variable with 2021 data.
– Europe: We refer to findings that include all available data as ‘Europe’. This generally refers to all EU countries except Germany, plus Switzerland, Iceland, Norway and the UK (annual data available until 2019). For some variables, income in particular, data is also missing for Sweden, Norway, and Iceland. Europe-wide figures are taking the average across all countries weighted by each country’s population or college-educated population.
Key Brain Waste Metrics
– Over-qualified: Working in occupations below one’s skill level. A typical example of an over-qualified worker would be a doctor who works as an assistant nurse. A person is over-qualified when their level of education is greater than is typical for a given occupation (specifically more than 1 standard deviation greater than the mean education level in their occupation, meaning that around 84% of people holding that occupation are less educated). This definition is based on the ‘statistical approach’ developed by Bauer 2002, Bonfanti and Xenogiani 2014, and Verdugo and Verdugo 1989.
– Under-employed: Not working as much as one wants to. For example, a part-time teacher is under-employed if they would like to and are able to work more. Respondents are under-employed when they work part time, “wish to work additional hours and are available to do so.” This follows the EU definition.
– Unemployed: Not working when one wants to work. This means that e.g., people with permanent disabilities that prevent them from working are not considered unemployed. The ELF uses the ILO definition of unemployment.
Other Labor Market Outcomes
– High-skilled: working in highly skilled professions. People working in occupations labelled as managers, professionals, technicians, and associate professionals are considered highly skilled. This follows the ISCO classification system.
– Mean income decile: The ELF only contains information about an individual’s monthly wage decile, i.e., what share of the population makes less than them. For example, a person who makes more than 35% of the population would be in the fourth decile. To estimate Euro values we joined the ELF to SES income data we obtained from Eurostat. The SES data contains earnings at each decile break, which means that it is an imperfect match to the mean income decile variable from the ELF (i.e., the ELF decile variable has 10 unique values while the SES has 9). To account for this imperfect match, we joined persons falling in the first and second earnings decile according to the ELF to the first decile break from the SES, people earning in the third and fourth decile to the third decile break, etc.
– Shortage occupation: An occupation with a lot of unfilled positions. An occupation is defined as a shortage occupation if it is included as a shortage occupation in this EURES report from 2022. Note that this report is based on 2022 data and data quality appears to differ between countries. Also, the occupation classifications in the report are more fine-grained than in the LFS (ISCO 4-digit rather than ISCO 3-digit). Any 3-digit occupation that includes a 4-digit occupation mentioned in the report is labelled as a shortage occupation. This means that we potentially overestimate the actual shortage on the 3-digit level because one 3-digit occupation group can contain multiple 4-digit occupations, not all of which may be affected by a shortage.
– Regulated profession: An occupation that requires some form of licence to perform. Any occupation mentioned in the EU’s Regulated Professions Database. The match between the database and the occupation classification in the Labor Force Survey is imperfect and was done manually. Our correspondence table between ISCO 3-digit occupations and the Regulated Professions Database can be found here. Regulated professions present a strong barrier to immigrants’ success in the labor market because their foreign credentials may not allow them to perform their profession in the host country. Migrants can seek to have their foreign credentials recognized, which is frequently touted as a solution to brain waste, and policies surrounding recognition have undergone substantial change in the past two decades, which we investigate in the Section ‘Recognition of Foreign Diplomas’.
– Fixed-term contracts: employment contract is limited in time. We do not distinguish between different contract lengths: as long as the contract contains an end date or duration, it would be measured as fixed-term.
THE SCALE OF BRAIN WASTE
Figure 1 compares all labor market outcomes based on combining 2017-2022 data. You can toggle between the outcome categories using the filter. ‘Native (mean)‘ and ‘Immigrant (mean)‘ are the shares of natives and immigrants to whom a particular labor market outcome applies. For example, 67.3% of college-educated immigrants in Italy are over-qualified for the positions they hold. ‘Raw difference‘ is calculated by subtracting ‘Native (mean)‘ from ‘Immigrant (mean)‘. ‘Controlled difference‘ is the impact of migrant status on labor market outcomes when holding constant other variables that are likely to impact labor market performance.
More technically, ‘Controlled Difference‘ is estimated using OLS regression with the following formula:

Where Y is the labor market outcome of respondent i in county c. MIGR is a binary variable indicating migrant status, X is a vector of control variables including, where available, respondent sex, age, age squared, number of children, marriage status, level of education, and time. In addition, beta 3 captures NUTS 2 region fixed effect. ‘Controlled Difference’ is the beta 1 coefficient and the significance level (appears on hover) refers to the same coefficient.
Key findings:
– Across Europe, with very few exceptions, immigrants are more likely to be over-qualified, more likely to be under-employed, more likely to be unemployed, less likely to work in high-skilled or regulated jobs, and earn less.
– In Italy, the ‘worst’ country when it comes to over-qualification, more than two-thirds of immigrants are over-qualified compared to around 41.3% of natives. The Europe-wide gap in over-qualification between immigrants and natives is greater than 14.6%, which means that immigrants are almost 45% more likely to be over-qualified for their jobs than natives.
– When it comes to under-employment, the gaps are much smaller (EU-wide, immigrants are under-employed at a rate that is around 1.2% greater than natives). But these small differences shouldn’t distract from the fact that very few people are under-employed to begin with (just over 2.1% of the workforce). This means that immigrants are actually under-employed at a rate more than 50% greater than natives. In some countries, the gap is substantially bigger. In Norway for instance, 3.9% of immigrants are under-employed, more than twice the native rate.
Figure 1a uses ‘Raw Difference’ from Figure 1, i.e., it reports the difference in means between native and immigrant labor market outcomes. Note that for some metrics, greater positive numbers indicate worse migrant outcomes (e.g., over-education), while for other metrics smaller negative numbers indicate concerning migrant native disparities (e.g., high-skilled jobs).
While the results differ slightly between labor market outcomes, a consistent pattern emerges: immigrants lag behind natives everywhere, but brain waste is worst in Southern Europe, Norway, and Sweden. This holds true when we look at over-qualification, under-employment, unemployment, and income (though most Scandinavian countries don’t report income figures). The one country that consistently bucks the trend in Southern Europe is Portugal where migrant-native gaps across all indicators are substantially smaller than elsewhere in the region.
France, Switzerland, Austria, Belgium, Ireland and Denmark tend to have smaller gaps between migrants and natives. The Netherlands and the UK frequently have the best labor market performance amongst immigrants compared to natives.
The data contained in Figure 1b is calculated the same way as in Figure 1. The key difference between the two is that Figure 1b is not restricted to college-educated respondents.
How does the inclusion of all immigrants affect the findings? With regard to over-qualification, two points stand out: first, over-qualification rates in the entire population are much lower than amongst the college-educated population. This is caused by the way we measure over-qualification, but it also makes intuitive sense – without education, it’s not possible to be over-qualified.
The other big difference to the college-only findings is that the raw differences in most Southern European countries is comparatively small or even negative. The reason for this is that the immigrant population in these countries is substantially less educated than the native population on average, making it more likely that natives are over-educated than immigrants, which is not the case elsewhere in Europe. Note that these negative differences reverse themselves in the ‘Controlled Difference’ column, where we hold constant respondents’ level of education.
Once we look at metrics of brain waste that are not dependent on education, such as under-employment and unemployment, the large gaps in Southern Europe (and Sweden) reappear. This indicates that these countries struggle to integrate migrants into the labor market in general, not just college-educated migrants.
Figure 2 is once again based on college-educated native and immigrant means and the raw difference between the two. The difference to previous graphs is that in Figure 2, the data is disaggregated by year. We did this to investigate whether brain waste became more or less severe over time. The differences in the time spans covered by the graphs above are caused by the fact that a number of variables are not available in some years or that the approach to collect them changed substantially at some point in time.
The clearest result from this analysis is that there are no strong patterns over time, especially when it comes to closing immigrant-native gaps in the labor market (‘Raw Difference‘). While unemployment or under-employment may ebb and flow in response to macro-economic developments (e.g., unemployment for the entire population increased in Greece during the Eurocrisis, while the rise in under-employment was concentrated amongst immigrants), the difference between both groups in most countries remains relatively constant over the past decade or so.
NOT EVERYBODY IS AFFECTED BY BRAIN WASTE IN THE SAME WAY
Global South vs Global North
For Figure 3, we calculated native means as before, but this time we divided up immigrants according to their country of birth into Global South (South America, Africa, Asia) and Global North (North America, Europe, Oceania). ‘Difference Global South-Global North’ is calculated by subtracting the ‘Global North migrants’ from the ‘Global South migrants‘.
Key findings:
– In most countries Global South immigrants are more affected by brain waste than Global North immigrants: they are over-qualified at higher rates (generally between 5% and 14% in most countries), under-employed more frequently (generally between 0.4% and 2.5%), more likely to be unemployed in every single country (between 0.4% and 7.8%), and more likely to have fixed-term contracts (between 2% and 10%).
– One frequent outlier in the data is once again Portugal: Global South immigrants in Portugal are 12.6% less likely to be over-qualified for their jobs, 0.8% less likely to be under-employed, and 8.4% less likely to have fixed-term contracts than Global North migrants. Global South migrants are 0.5% more likely to be unemployed than Global North migrants, but this is the smallest difference of any country. Similarly, in France, Global South immigrants do comparatively well but the numbers are less striking than for Portugal (+0.9% for over-qualification, -0.5% for under-employment, 2.8% for unemployment, and +2.3% for fixed-term contracts).
Gender Breakdown
For Figures 4a and 4b, we broke down the results by gender. Figure 4a contains the means of the 2017-2022 data for native men, native women, immigrant men, and immigrant women. Deselect individual groups by clicking on the legend.
Figure 4b looks at immigrant-native differences for men and women separately, and compares the size of the differences. More precisely ‘difference in gap between men and women’ = ‘women: migrants – natives‘ – ‘men: migrants – natives’. Finally, ‘regression’ is the coefficient on the interaction effect between gender and migration status (otherwise the regression equation is the same as Equation 1).
From Figure 4a, it becomes clear that native men and women each do better and worse in different labor market outcomes: men are more likely to be over-qualified and less likely to work in a regulated profession, but they are more likely to earn more, less likely to be under-employed, and more likely to work in a high-skilled job than women.
Gender-differences for unemployment rates differ by country. While the results are a little bit less clear cut for immigrants, especially for over-qualification, most gendered employment outcomes are remarkably similar amongst both natives and immigrants.
Key findings:
– With few exceptions, brain waste is affecting both men and women. In other words, both migrant men and women have worse labor market outcomes than native men and women, respectively.
– Women are affected more than men by brain waste when it comes to over-qualification, under-employment, and unemployment (the findings are less consistent for income). In other words, the native-immigrant gap is greater for women than for men. In addition, in countries where the male immigrant-native gap is greater for men than the women, fewer of the results are statistically significant.
– There are a few countries that stand out and maybe unsurprisingly they tend to be Nordic. Female immigrants in Finland are less affected by over-qualification and under-employment than male immigrants (though both findings are not statistically significant). Meanwhile in Iceland and Sweden, female immigrants are less affected by unemployment (and this time, the results are significant).
Regional Breakdowns
Brain waste isn’t evenly distributed across countries. To explore sub-national differences in employment outcomes, we grouped the data by NUTS 2 regions (the smallest geographical region available for most countries in the ELF), and calculated immigrant and native labor market outcomes, as well as differences between the two (once again taking the average across the 2017-2022 data).
Key findings:
– Over-qualification differences between immigrants and natives are concentrated in Flanders, Northern Italy, the Peloponnese and the Cyclades, in North-Eastern Spain as well as in Silesia.
– Under-employment differences are concentrated in Normandy, Poitou-Charentes, Extremadura, and Sterea Ellada.
-Unemployment differences are once again concentrated in Extremadura, Western Greece, as well as Macedonia.
– Finally, fixed-term contracts are much more common for immigrants than natives throughout Poland and on the Cycladic islands.
Breakdowns by Occupation and Education
The next three graphs explore how brain waste intersects with fields of education and occupation. By analyzing educational backgrounds of migrants, we can see which education backgrounds are less of an impediment to finding appropriate work and which still present a major barrier to immigrants compared to natives. Occupation gives an idea of where migrants who are not able to find work appropriate for their education end up working instead.
Figure 6 shows the raw immigrant-native difference in brain waste outcomes grouped by field of education.
Key findings:
– Education stands out as a field where immigrants are much more likely to be over-qualified than natives. In Greece, this gap is a striking 37.1%. The one country that does not fit this trend is France, where immigrants are actually 5.6% less likely to be over-qualified than natives.
– When it comes to under-employment amongst educators, France’s gap is once again below zero. But in Switzerland and the UK, immigrant educators are over 4% more likely to be under-employed than their native counterparts.
– With regards to unemployment, France stands out in the opposite direction: immigrant educators in the country are over 4% more likely to be unemployed than their native counterparts. The number is similar in Italy and Sweden. In Finland the difference stands at 8.4%, showing the difficulty the country has in integrating migrant teachers.
– For immigrants with health care degrees, there are substantial disparities across Europe. While immigrants are over-qualified at essentially the same rate as natives in France, the Netherlands, and the UK, they are over 20% more likely to be over-qualified in Italy, and the gap is 16.3% in Belgium. The immigrant-native differences for under-employment are relatively small; Sweden and Norway, however, stand out because immigrants are under-employed at rates 1.5% greater than natives. Differences in unemployment are most concentrated in Greece (4.3%), Spain (3.8%), and Sweden (3.7%).
Figure 7 shows shares of immigrant and native populations broken down by specific ISCO 3-digit occupations as well as gender. We chose to show shares of population in this graph because college-educated respondents will, by definition, be over-qualified for some jobs (e.g., janitorial roles) or not over-educated for others (surgeons).
There is a lot to explore in Figure 7 (filter by job using the dropdown menu). For almost every job there are substantial differences by gender and migration status. The graph links to a dataset that allows you to explore those metrics too.
Key findings:
– Medical doctors: In a number of Northern and Western European countries, e.g., the UK, Ireland, France, and Norway, college-educated immigrants are much more likely to be doctors than natives, and men are much more likely to be doctors than women. Switzerland and Denmark are interesting because, once again, immigrants are more likely to be doctors, but here women rather than men dominate the field. On the other end of the spectrum, there are some countries, such as Italy, where men and natives dominate the profession.
– College-educated immigrants are also much more likely to occupy lower paying jobs, like cooks, builders, or bus and truck drivers.
– Other service jobs are dominated by immigrant women, for instance shop sales persons or personal care workers (in Italy, 15.1% of immigrant women work in personal care compared to just 0.8% of native women).
We created Figure 8 primarily to explore the intersection of education and brain waste. The data shown is for immigrants only and brain waste metrics are calculated for each field of education-occupation pair separately. This helped us identify professions in which, e.g., teachers who work below their skill level end up working, and informed our ground reporting.
THE COSTS OF BRAIN WASTE
Brain waste has a personal cost to each migrant who is impacted and to the overall economy of the country that is wasting immigrant talent. It is difficult to estimate the exact economic cost of brain waste because there are a number of second order effects that might occur if brain waste was eliminated. For example, more immigrants entering the labor market might depress native wages. On the other hand, adding workers to the economy could allow it to grow dynamically, making everyone better off in the end. It is unclear whether eliminating or reducing brain waste would lead to positive or negative secondary consequences for the larger economy.
Rather than trying to estimate such ‘equilibrium outcomes’, we calculated the economic costs of brain waste using two relatively straightforward scenarios, both of which essentially assume there there are unlimited jobs: the first one is based on the question “how much could immigrants (and by extension the economy) gain in wages if immigrants were earning the same as natives?” We call this the ‘basic wage model’ and it is estimated using OLS regression with the following equation:
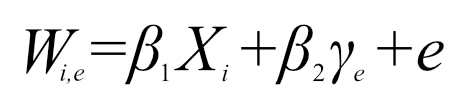
where wages W of individual i in NUTS 2 regions are estimated using a set of predictor variables including, where available, sex, age, age squared, number of children, marital status, and level of education as well as region fixed effects. We estimated this model for natives using 2020 data only, the last year for which ELF income data is available.
With this model in hand, we ‘predicted’ how much an immigrant would make if they were earning the same annual wages as a comparable native, i.e., a native with the same age, gender, place of residence, etc. In fact, most immigrants make substantially less. The average difference between the two numbers is reported in the ‘basic model‘ column of Figure 9.
The second model, ‘wage model controlling for education‘, is calculated in exactly the same way as the first, with the only difference that ‘field of education’ is now introduced as an additional control variable (we chose to calculate both models because ‘field of education’ is frequently missing).
The remaining two models contain two steps. First, we figured out how much less under-employed and over-qualified natives make than appropriately employed natives. To do this, we used the same wage model as described in Equation 2, with the addition of over-qualification or under-employment as predicting variables:

Intuitively, the beta 1 coefficient captures the over-qualification/under-employment penalty – the personal annual wage loss of working in a job below one’s skill level or involuntarily working part-time. These penalties are in the columns ‘over-qualification penalty‘ and ‘under-education penalty’.
Assuming the same wage penalty for immigrants, we then calculated how much more immigrants could be earning nation-wide if their over-qualification or under-employment rates declined to native levels.
These aggregate results can be seen by clicking on ‘national lost wages (EURO)‘ in the dropdown menu. In this setting, the ‘basic wage model‘ and ‘wage model controlling for education’, similarly show how much immigrants could earn in additional wages if they earned at levels comparable to natives.
This analysis excludes Norway, Sweden, and Iceland because individual income data was removed from the ELF for these countries. This also means that these countries are excluded from the Europe-wide figures.
Key findings:
– In all countries except Switzerland and some Eastern European countries, immigrants would earn substantially more than they currently do if they were paid the same as natives. Controlling for education, the EU wide average is 1835 Euros per person. Similarly over-qualification and under-employment are associated with substantial wage penalties.
– Once again, controlling for education, we estimate that the European economies for which we have data could grow by 33.8 billion Euros (or 0.36% of annual GDP) if immigrants earned the same as natives. Not all of this lost income is caused by over-qualification and under-employment, but if immigrant over-qualification and under-employment rates declined to native levels, European economies could gain 2.4 and 4.2 billion Euros respectively (or 0.03% and 0.05% of annual GDP).
These numbers are very rough estimates. It would be difficult for immigrant labor market performance to fully approach native levels even if all discrimination were eliminated (see Section ‘The Causes of Brain Waste’). Still, this analysis makes clear that brain waste, and under-employment and over-education in particular, come at substantial costs to Europe’s economies.
THE CAUSES OF BRAIN WASTE
Education and Language
Figures 10-14 are constructed in more or less the same way. The country-level data is first restricted to 2017-2022 or just 2021, depending on the availability of data. Then the data is grouped according to an immigrant characteristic, e.g., where immigrants were educated, and for each group, mean labor market outcomes are calculated.
In addition, we estimated labor market outcomes for different immigrant subgroups using the same set of controls as explained in Equation 1. ‘Natives’ always serve as the reference category for these regressions. This analysis tells us if a given group, e.g., immigrants educated abroad, have labor market outcomes that differ significantly from natives. The significance level of these regressions are shown on hover, the controlled differences are available in the underlying datasets.
Key findings:
– In almost all countries for almost all metrics, immigrants educated abroad perform substantially worse in the labor market than immigrants educated domestically, i.e., within the host country, as well as natives. EU-wide, foreign-educated immigrants are over-qualified at a rate of around 54.5 % compared to 40.6% amongst domestically educated immigrants and 32.9% amongst natives. The results are relatively similar for both under-employment and unemployment.
– Portugal is once again an interesting example, where the place of education appears to matter even more: domestically educated immigrants are only 3.2% more likely to be over-qualified and actually less likely to be under-employed or unemployed than natives (the latter two results are not statistically significant, however).
– Luxembourg and Switzerland stand out as countries where foreign education is not associated with greater rates of over-qualification than domestic education. This might be caused by the exceptional employment opportunities relative to their countries of origin for skilled knowledge workers.
Europe-wide, immigrants with better language skills have better employment outcomes. In 2021, immigrants with poor host country language skills were over-qualified and under-employed at almost twice the native rate and unemployed at more than double the native rate (Figure 11).
While immigrants who did not take a language course are more likely to be over-qualified than those who did, who in turn are more likely to be over-qualified than immigrants who are proficient in the host country language, the results for under-employment and unemployment are more surprising (Figure 11a).
In Finland and Spain, immigrants who took a language course are actually more likely to be under-employed and/or unemployed than those who did not. However, it is likely that a language class is seen as a pathway to improved labor market outcomes for immigrants who are dissatisfied with their current jobs. Unfortunately, because the ELF is a cross-sectional survey, we cannot directly test whether language courses actually work to improve labor market outcomes, but Figure 11 strongly suggests that they do.
Time in the Country and Reason for Migration
Pretty consistently, immigrants who spend more time in the country experience better labor market outcomes. This makes intuitive sense and is backed up by a host of academic research. It takes time to acclimate, build up one’s network, and learn host country specific skills, especially language. One exception to this pattern is Greece, where long-term immigrants are actually more likely to be unemployed or under-employed than more recent immigrants.
Across Europe, asylum seekers have the worst labor market outcomes of any immigrant group. It is important to note here that in many European countries, asylum seekers cannot legally work, especially in the initial stages of their procedure. Academics have found these early restrictions tend to hurt refugees’ labor market performance in the long-run. These restrictions can likely account for a large portion of the pattern in Figure 13.
In Scandinavian countries, as well as Spain and Ireland, immigrants who came to the country for education are under-employed at higher rates than any other immigrant group.
Recognition of Foreign Diplomas
The non-recognition of foreign diplomas is often mentioned as a reason for brain waste. In the words of the European Commission, eased access to diploma recognition “has a fundamental role to play in easing skills shortages and mismatches, which have an impact on the lives of individuals, the profitability of enterprises, and the capacity for growth and prosperity of the European economy as a whole”. Two studies, one based in Israel and another from Germany, largely agree with this assessment – both find that recognition of foreign diplomas substantially improves immigrants’ labor market outcomes.
Key findings:
– Immigrants who have sought and received diploma recognition are over-qualified at much lower rates (38.5%) than immigrants who have not (56.2%), across Europe.
– Interestingly, these results don’t translate to under-employment and unemployment. For both of these metrics, there are a number of countries across Western and Northern Europe, where immigrants who have sought recognition do worse than those who have not. As with language courses, this may be a case of mistaking cause and effect: a bad job may be what prompts immigrants to seek recognition of their foreign diplomas. Alternatively, it could be the case that jobs for which recognition is required (regulated professions like doctors, lawyers) are systematically more hostile to immigrants, diploma recognition notwithstanding. It is not possible to tell which is the case without access to longitudinal data, which unfortunately we do not have.
Diploma non-recognition is one of the barriers immigrants face in the labor market with a very straight-forward policy solution: make it easier for immigrants to obtain the proper accreditation to perform their job in the host country. Indeed, the EU and a number of member states did exactly this and Figure 15 explores whether these policy changes had their intended effect. Specifically, we were interested in the impact of three policies:
– Directive 2005/36/EC, which made recognition of degrees for architects, dentists, doctors, midwives, nurses, pharmacists, and veterinary surgeons automatic and was meant to streamline recognition procedures for everybody else. This directive had to be implemented in national law by October 2007. Only EU/EEA immigrants were affected by this policy.
– Directive 2013/55/EU, which expanded on Directive 2005/36/EC. Only EU/EEA immigrants were affected by this policy.
– National overhauls of diploma recognition procedures in Spain and Italy implemented 2014 and 2009, respectively. All immigrants were affected by this policy.
To test the impact of these policies we used a difference-in-differences estimator. The intuition behind this approach is to compare a treatment group (immigrants to whom the policy change applies) with a control group (immigrants to whom the policy change does not apply or natives) both before and after the policy was implemented. The estimator tells us whether the gap between the two groups has changed after the policy was implemented. In other words, it detects a trend break. For example, we would expect that after Directive 2005/36/EC went into effect, the rates of over-qualification, under-employment, and unemployment rates among EU/EEA immigrants with relevant degrees to drop compared to the rates for migrants for whom the directive did not apply, those not from EU/EEA.
We estimated the effect of the policy changes with the following equation:

Where Y is the employment outcome of individual i in NUTS 2 region c. Beta 1 captures the interaction effect of the treatment and a binary variable for the post period. We used the same controls as elsewhere, including sex, age, age squared, marital status, level of education, number of children, time, and NUTS 2 regional fixed effects.
Figure 15 shows the beta 1 coefficient for the different policies which are color coded. The drop down can be used to change the employment outcome and shapes indicate whether the effect is statistically significant at the P < 0.05 level or not (crosses indicate significance). The left chart looks at whether the policies affected labor market outcomes of EU immigrants relative to non-EU (TCN) immigrants, the center chart compares EU immigrants with natives, and the right chart compares TCN with natives (this comparison is only relevant for the country specific policies in Italy and Spain).
The main takeaway from this graph is that the policy changes do not seem to have a systematic impact on immigrant labor market outcomes. In some countries unemployment or under-employment rates went up, in others they went down (if the policies changed immigrant’s labor market prospects for the better, we would expect to find consistently negative effects). For some countries the results are significant, for others they are not. A lot of the results are very close to zero.
Even for regulated professions, there is no systematic impact. Recognition of foreign diplomas is usually required to work in a regulated profession such as nursing, but it does not appear that after the policies were passed more immigrants entered regulated occupations. Overall then, it looks like the policies did little to help immigrants succeed in the labor market.
Does this mean the policy changes were entirely ineffective? Not necessarily. For instance, after Germany passed a comprehensive reform of its recognition regime, more people applied to have their degrees recognized. And, as Figure 14 shows, degree recognition is at the very least associated with a reduction in over-qualification. It just looks like diploma recognition is not the silver bullet, it is made out to be since we do not see large shifts in labour outcomes for college-educated migrants.
There are, however, a number of limitations to this analysis. The EU policies in particular targeted specific occupations, for which recognition became automatic. Unfortunately, the sample sizes of immigrants who had previously worked in these professions in their country of origin were too small to reliably say whether the policies had any of the intended outcomes. So it is possible that there is a smaller subset of immigrants for whom the policies had their intended effect.
It is also quite possible for the impact of the policies to manifest several years after they were passed. We checked whether this is the case but did not find a systematic pattern. We also tried to establish whether the treatment and control groups actually moved in parallel, prior to the policies’ implementation, with very mixed results. In other words, there are several trend breaks in the data, when some immigrants’ labor market outcomes improve relative to native or other immigrants, but these trend breaks appear to have little to do with the policy changes.
Finally, easier access to diploma recognition (and thus high paying jobs) may attract more qualified immigrants (visas are also frequently linked to prior recognition). Had we found any strong effects of the policies, we would have needed to make sure that any improvements in labor market outcomes aren’t explained by compositional changes in the immigrant population. Since we did not find such effects, all we can say is that Figure 15 more is likely still an over-estimation of the policies’ impact.
Discrimination
Discrimination is not included in our analysis simply because a whole host of studies have done an outstanding job showing the persistence of discrimination against immigrants, particularly non-white immigrants throughout European labor markets. In a meta-analysis, a study that combines the findings of many individual studies, Lincoln Quillian and his coauthors find discrimination in every single labor market they studied.
MATCHES BETWEEN SHORTAGES AND BRAIN WASTE
The most straightforward solution to “solving” brain waste is for more immigrants to find employment that corresponds to their skills and desire to work. Most European economies have substantial shortages across a number of sectors. Figure 16 explores whether immigrants could be “matched” to better employment opportunities in such fields.
We calculated shortages using the share of natives who work in a shortage occupation, according to the 2022 EURES report, by field of education. Immigrant under-employment, over-education, and unemployment are calculated in the same ways as in Figures 6-8.
Key findings:
– Education: there are large teacher shortages across Europe. In Norway, the Netherlands, Switzerland, and Belgium, the share exceeds 90%. Despite this, immigrants with education degrees are frequently over-qualified for their jobs, which usually means that they don’t work as full teachers, often exceeding 30% or even 40%.
– Health: the shortage picture for healthcare is similarly dire to education. There are still a number of countries with high over-qualification rates, such as Greece, where, according to the EURES report, there is not a shortage of healthcare workers. In Italy and Ireland, there are moderate shortages of healthcare workers, yet immigrants are still over-qualified at more than 35%. Other countries seem much more able to integrate immigrant health care workers into their work force in appropriate roles: Finland and Denmark stand out in this regard with over-qualification rates of only 18.2% and 21.7%, respectively.
LIMITATIONS
While most variables are available for most years and countries, sometimes the exact way questions were asked differs by country or time. We harmonized variables as much as possible, but it is possible that we aren’t aware of some national idiosyncrasies. There are also important differences in the sampling approach used in different countries. Sample sizes in Central and Eastern countries were frequently too small to report them here and even after aggregating the results to country groups (see ‘Preprocessing’ for more details), one should be careful to draw strong conclusions from the data we present.
For regression analysis in particular, we set up the analysis in such a way that if a given variable is missing for a particular country, it simply wouldn’t be used as a control. In other cases, so few respondents fall into a particular category that we couldn’t report the results without violating Eurostat anonymization criteria. We used 50 respondents as our lower cutoff for reporting results. We did not use sample weights for either the descriptive or the regression analyses.
Most importantly, this is a cross-sectional survey, which means that we cannot compare respondents over time. For our project, this means that we always need to be aware that changes in labor market outcomes could be explained not by actual developments in immigrants’ labor market performance but by changes in the immigrant population as such, e.g., an influx of highly educated individuals due to a special visa program.
ATTRIBUTION
This report is based on data from “Eurostat. 2024. “EU – Labour Force Survey Microdata 1983-2022, Release 2023.” https://doi.org/10.2907/LFS1983-2022.” The responsibility for all conclusions drawn from the data lies entirely with the Lighthouse Reports and its media partners.